일 | 월 | 화 | 수 | 목 | 금 | 토 |
---|---|---|---|---|---|---|
1 | 2 | 3 | 4 | 5 | 6 | |
7 | 8 | 9 | 10 | 11 | 12 | 13 |
14 | 15 | 16 | 17 | 18 | 19 | 20 |
21 | 22 | 23 | 24 | 25 | 26 | 27 |
28 | 29 | 30 |
- Deep learning
- AI
- 기계학습
- GAN
- meta-learning
- 코딩 테스트
- grad-cam
- keras
- 설명가능한
- Unsupervised learning
- 딥러닝
- 메타러닝
- SmoothGrad
- 설명가능한 인공지능
- xai
- python
- coding test
- Class activation map
- 시계열 분석
- Score-CAM
- 백준
- 머신러닝
- Explainable AI
- Artificial Intelligence
- 코딩테스트
- 인공지능
- Machine Learning
- cs231n
- Cam
- Interpretability
- Today
- Total
목록GAN (6)
iMTE
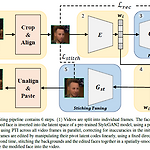
논문 제목 : Stitch it in Time: GAN-Based Facial Editing of Real Videos 논문 주소 : https://arxiv.org/abs/2201.08361 Youtube video : https://www.youtube.com/watch?v=4lQkQSmA8nA Stitch it in Time: GAN-Based Facial Editing of Real Videos The ability of Generative Adversarial Networks to encode rich semantics within their latent space has been widely adopted for facial image editing. However, replicating th..
Pix2PixImage-to-Image Translation with Conditional Adversarial NetworksIsola, Phillip, et al. "Image-to-image translation with conditional adversarial networks." arXiv preprint (2017). cycleGAN, DiscoGAN, Pix2Pix 와 같은 image-to-image translation model은 보란듯이 첫 페이지에 결과를 보여준다. (이러니 안궁금할 수가 없지.) 핵심만 간단하게 해석하고 접근을 해보자.먼저, Pix2Pix는 다른 cylceGAN, DiscoGAN과 달리 Paired image를 요구한다. 즉, input output이 서로 관련이 있..
DiscoGANLearning to Discover Cross-Domain Relations with Generative Adversarial NetworksKim, Taeksoo, et al. "Learning to discover cross-domain relations with generative adversarial networks." arXiv preprint arXiv:1703.05192 (2017).GAN에서 직접적으로 결과를 보여줄 수 있는 것은 역시나 이미지인 것 같다. cycleGAN도 그렇고, 이미지로 확 impact를 주니.. 안궁금할 수가 없는 것 같다. cycleGAN과 굉장히 유사한 개념을 기반으로 loss function을 정했고, network를 구성했다. 이 논문에서 cy..
CycleGANUnpaired Image-to-Image Translation using Cycle-Consistent Adversarial NetworksZhu, Jun-Yan, et al. "Unpaired image-to-image translation using cycle-consistent adversarial networks." arXiv preprint (2017).위의 그림에서 보는 것과 같이, cycleGAN은 서로 다른 domain의 이미지를 translate하는 'Image-to-Image translation' GAN이다. GAN이라는 단어가 사용되었기 때문에 당연히, Discriminator와 Generator는 서로 'Adversarial learning'을 시행한다. Cycle..
Super-resolution GAN (SRGAN)Photo-Realistic Single Image Super-Resolution Using a Generative Adversarial NetworkLedig, Christian, et al. "Photo-Realistic Single Image Super-Resolution Using a Generative Adversarial Network." CVPR. Vol. 2. No. 3. 2017. 간략하게 설명하자면, 기존의 super-resolution 문제에 대해서 단순히 MSE(Mean squared error)를 loss function(objective function)으로 정해놓고 optimization을 해본 결과, high peak sign..
Generative Adversarial Networks (GAN) 두 신경망이 '경쟁'하면서 서로 학습하는 재미있는 아이디어를 가진 network를 Generative adversarial networks 라고 부른다. 두개의 신경망 중 하나는 1. Generative model, 2. Discriminator모델이다. 흔히 이 모델을 설명할 때 위조지폐를 만드는 범인과 이를 감독하는 경찰의 예를 든다. 경찰은 범인이 만든 위조 지폐를 구분을 하고, 거짓인지 진짜인지를 밝힌다. 범인은 이런 과정을 보고 경찰을 속이기 위해서 더 나은 위조 지폐를 만들어 낸다. 그러면 경찰은 다시 더 정밀하게 구분하도록 학습을 하게되고.. 최종적으로는 경찰은 위조 지폐를 분류할 거짓이라고 확률이 50 %가 될 정도로 정밀..