일 | 월 | 화 | 수 | 목 | 금 | 토 |
---|---|---|---|---|---|---|
1 | ||||||
2 | 3 | 4 | 5 | 6 | 7 | 8 |
9 | 10 | 11 | 12 | 13 | 14 | 15 |
16 | 17 | 18 | 19 | 20 | 21 | 22 |
23 | 24 | 25 | 26 | 27 | 28 | 29 |
30 | 31 |
- 코딩테스트
- Cam
- Deep learning
- cs231n
- 설명가능한
- 코딩 테스트
- AI
- 기계학습
- Explainable AI
- 딥러닝
- Artificial Intelligence
- Class activation map
- keras
- 설명가능한 인공지능
- 백준
- Machine Learning
- coding test
- 시계열 분석
- grad-cam
- 인공지능
- Interpretability
- 머신러닝
- xai
- GAN
- Unsupervised learning
- 메타러닝
- meta-learning
- SmoothGrad
- Score-CAM
- python
- Today
- Total
목록Gradient (3)
iMTE
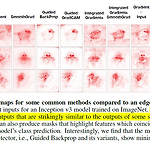
논문 제목 : Sanity checks for saliency maps 논문 주소 : arxiv.org/abs/1810.03292 Sanity Checks for Saliency Maps Saliency methods have emerged as a popular tool to highlight features in an input deemed relevant for the prediction of a learned model. Several saliency methods have been proposed, often guided by visual appeal on image data. In this work, we propose an a arxiv.org 주요 내용 : 1) Saliency map은 학..
논문 제목 : Sanity checks for saliency maps 논문 주소 : arxiv.org/abs/1810.03292 Sanity Checks for Saliency Maps Saliency methods have emerged as a popular tool to highlight features in an input deemed relevant for the prediction of a learned model. Several saliency methods have been proposed, often guided by visual appeal on image data. In this work, we propose an a arxiv.org 주요 수식 정리: 0) Definition in..
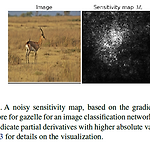
논문 제목 : SmoothGrad : removing noise by adding noise 논문 주소 : arxiv.org/abs/1706.03825 SmoothGrad: removing noise by adding noise Explaining the output of a deep network remains a challenge. In the case of an image classifier, one type of explanation is to identify pixels that strongly influence the final decision. A starting point for this strategy is the gradient of the class score arxiv.org 주요 ..