일 | 월 | 화 | 수 | 목 | 금 | 토 |
---|---|---|---|---|---|---|
1 | ||||||
2 | 3 | 4 | 5 | 6 | 7 | 8 |
9 | 10 | 11 | 12 | 13 | 14 | 15 |
16 | 17 | 18 | 19 | 20 | 21 | 22 |
23 | 24 | 25 | 26 | 27 | 28 | 29 |
30 | 31 |
- 설명가능한 인공지능
- SmoothGrad
- coding test
- 설명가능한
- Artificial Intelligence
- Class activation map
- Deep learning
- 기계학습
- 딥러닝
- 머신러닝
- 코딩 테스트
- Cam
- keras
- grad-cam
- GAN
- Machine Learning
- python
- cs231n
- Score-CAM
- Unsupervised learning
- xai
- 시계열 분석
- 백준
- 인공지능
- 메타러닝
- Explainable AI
- Interpretability
- 코딩테스트
- meta-learning
- AI
- Today
- Total
목록deep neural network (2)
iMTE
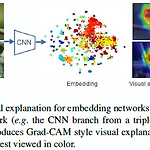
논문 제목 : Adapting Grad-CAM for Embedding Networks 논문 주소 : https://openaccess.thecvf.com/content_WACV_2020/html/Chen_Adapting_Grad-CAM_for_Embedding_Networks_WACV_2020_paper.html WACV 2020 Open Access Repository Lei Chen, Jianhui Chen, Hossein Hajimirsadeghi, Greg Mori; Proceedings of the IEEE/CVF Winter Conference on Applications of Computer Vision (WACV), 2020, pp. 2794-2803 The gradient-weighte..
Deep neural network는 대표적인 black box로 내부에 어떤 경과를 통해서 decision이 되었는지를 알지 못하는 문제가 있다. Support Vector Machine도 그런점을 갖고 있다. 반대로 Decision tree는 decision 과정을 확인할 수 있기 때문에 대표적인 white box 형태의 machine learning model이다. Deep neural network는 output layer의 activation 결과를 바탕으로 learned filter가 어떤 feature map을 만들었는지를 확인할 수 있다. 다음은 pytorch로 pre-trained vgg16의 feature map을 확인한 결과이다. 그리고 learned된 filter의 형태를 나타냈다.